This newly released article highlights some of the important elements of working holistically with your measurement framework. We have highlighted the most important parts and added Annalect’s view to this article.
Changes in the digital advertising industry, including Apple’s restrictions on tracking user data. As a result, deterministic user-level measurement of digital advertising effects is becoming more difficult. Businesses that fail to adapt to this new environment may lose valuable insights. In contrast, marketing mix models (MMMs) offer a distinct advantage because they can provide reliable measurements and insights without requiring user-level data, relying solely on natural variations in aggregate data.
The article discusses the advantages of using marketing mix models (MMMs) for digital ad measurement. With Apple’s new restrictions on tracking user data, MMMs are making a comeback because they can produce dependable measurements and insights purely from natural variations in aggregate data, without the need for user-level data. However, MMMs can be imprecise and misinform marketing decisions, especially when highly personalized ad campaigns are used on digital channels. To alleviate this, ad experiments are needed to calibrate MMMs.
The article provides three ways to calibrate MMMs using ad experiments and highlights the importance of calibration via ad experiments. The experiments can focus on on-site outcomes or use differential privacy to match off-site outcomes with on-site behavior. Geo ad experiments, where consumers in certain geographic regions see experimental ad campaigns while others do not, are used to measure the incremental impact of the experimental ads. Companies that rely on just a few channels and smaller brands with niche market segments may need to run experiments more frequently to refine their MMMs.
The article highlights some specific areas to improve the MMM results, which we in Annalect find relevant and part of the ongoing discussions you a MMM vendor should have with your clients:
-
- Use ad experiments to calibrate MMMs: As the article suggests, brands should use ad experiments to calibrate their MMMs to alleviate potential imprecisions in estimates. Ad experiments can help brands refine their MMM through experimental calibration, which can help them feel more confident in the information the model is giving them. Brands should compare the results of MMM and ad experiments to ensure that they are similar and use experiment results to choose between models.
- Focus on geo ad experiments: With the decrease in the ability to deterministically observe user behavior across websites and apps, brands should focus on geo ad experiments. In geo ad experiments, consumers in certain geographic regions see experimental ad campaigns, while consumers in others do not. Differences in sales and brand recognition between exposed and non-exposed geo units are used to measure the incremental impact of the experimental ads. This approach can provide a ground truth to calibrate the MMM against.
- Consider technologies such as differential privacy: Brands can also consider technologies such as differential privacy to match information between different datasets without revealing information about individuals. Randomization induced on one app/website in one dataset could then be matched to outcomes such as purchases observed on another app/website in another dataset.
- Refine MMMs for highly personalized ad campaigns: Highly personalized ad campaigns, as are often used on digital channels, can make it difficult to use MMMs effectively. Refining MMMs through experimental calibration, guided by a well-understood measurement plan, can help brands account for attentional and competitive dynamics that vary a lot across ad channels.
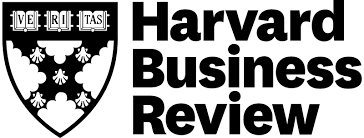
Read the full article here: https://hbr.org/2023/03/a-new-gold-standard-for-digital-ad-measurement